Our Case Studies
- Case #1 : Authorship Cheating: Helping to Confirm a Student’s Authorship
- Case #2 : Authorship Determination: Helping to Identify Source of Ananymous Hate Speech
- Case #3 : Authorship Determination: Helping with Reputation Management Issues
- White Paper: Linguistic Fingerprinting to Flag AI Authorship Cheating
CASE #1 : AUTHORSHIP CHEATING: HELPING TO CONFIRM A STUDENT’S AUTHORSHIP
Nancy was sitting in her office, scratching her head. She was a professor at one of the premier business schools in the country. For over 20 years she had been teaching Management 601, a management introduction class. Each year she faced a similar dilemma, how to identify and weed out those papers that were not written by the student who claimed them as their own. As the online world advanced, and with it, access to sophisticated service providers around the world, she was suspicious that the quantity, and quality, of these contract cheaters was ever rising. Were her students contracting others to author papers on their behalf?
Over the weekend, she was having dinner with a family friend, who is a leading expert in the field of forensic linguistics, told her of a new authorship identification technology that his company, Flint AI, was working on. The technology applies a combination of rules of linguistics, statistical analysis and software algorithms to analyze a document and determine the likelihood that the document indeed was written by the author.
The following day a meeting was scheduled for the school’s administrative integrity department to which Nancy had just recently been appointed. As a researcher, Nancy was intrigued by the potential of leveraging forensic linguistics and decided to engage in a systematic comparative approach to evaluate a possible solution to her dilemma.
The next day, upon Nancy’s recommendation, the committee commissioned a trial. An expert in forensic linguistics, one who was well known, and provided linguistics analysis for many federal agencies, was retained to evaluate a submitted paper that was suspicious. The following week, the expert submitted their analysis.
In the report, the expert explained their process in detail leveraging the science of forensic linguistics. Their analysis was that the paper at question was written by a foreign native speaker graduate student, one to whom English was a foreign language. Nancy noted that there was a sharp difference between the papers submitted by this student over the course of a couple of semesters. Aware that her writing skills were not sufficiently proficient, the student admitted to having retained a service to edit her English, specifically the grammar, however she was adamant that she wrote the paper on her own. Moreover, she claimed that she did not submit that paper as it was edited by the 3rd party service, because she thought it was not good enough, but rather submitted the written paper, as she wrote it originally.
At that point six additional papers from the student were then submitted to the expert. These were papers that were written in class, of which the student was the unquestioned author. The expert created a collection of documents on similar topics, and also reviewed papers from the web site that Nancy suspected the student was using for the alleged cheating authorship.
The expert analyzed over 16,000 words in the documents where the student was the known author. In the analysis, the expert parsed the documents to their linguistic components including: looking for word patterns; recurring spelling errors; recurring punctuation patterns; and sentence structure.
The conclusion was that the student indeed authored the suspect document. The expert opined that the consistency of the frequency of linguistic patterns and errors between the suspect document and the known documents supported the conclusion that indeed she was the author. The expert expressed their opinion that the difference and progress in the language skills was a natural progression of a skilled and studious student who was looking to improve her writing skills.
Time spent on the analysis alone (not including meeting) 20 hours. The cost of the analysis – over $1,200.
In the meantime, Nancy ran her own comparative evaluation. The same documents were submitted to the Flint AI Authorship Identification software program. The next morning a report was in Nancy’s in-box. The results – nearly identical.
Time spent: computer time measured in minutes. Cost – under $20.
Result: a studious and conscientious student was cleared of any suspicion of contract cheating and was not thrown out of a graduate academic program.
At their next meeting, Nancy and the committee discussed the findings. With the proliferation of contract cheating, they concluded that they clearly could not retain an expert to perform a manual analysis of each and every case of suspect contract cheating. They also discussed the potential of using the Flint AI software. Although is was generally recognized that it couldn’t be used as a final adjudicator, they did see that it could serve as a viable tool for initial screening, producing reliable timely cost effective results that would indicate whether follow up action with human intervention is required.
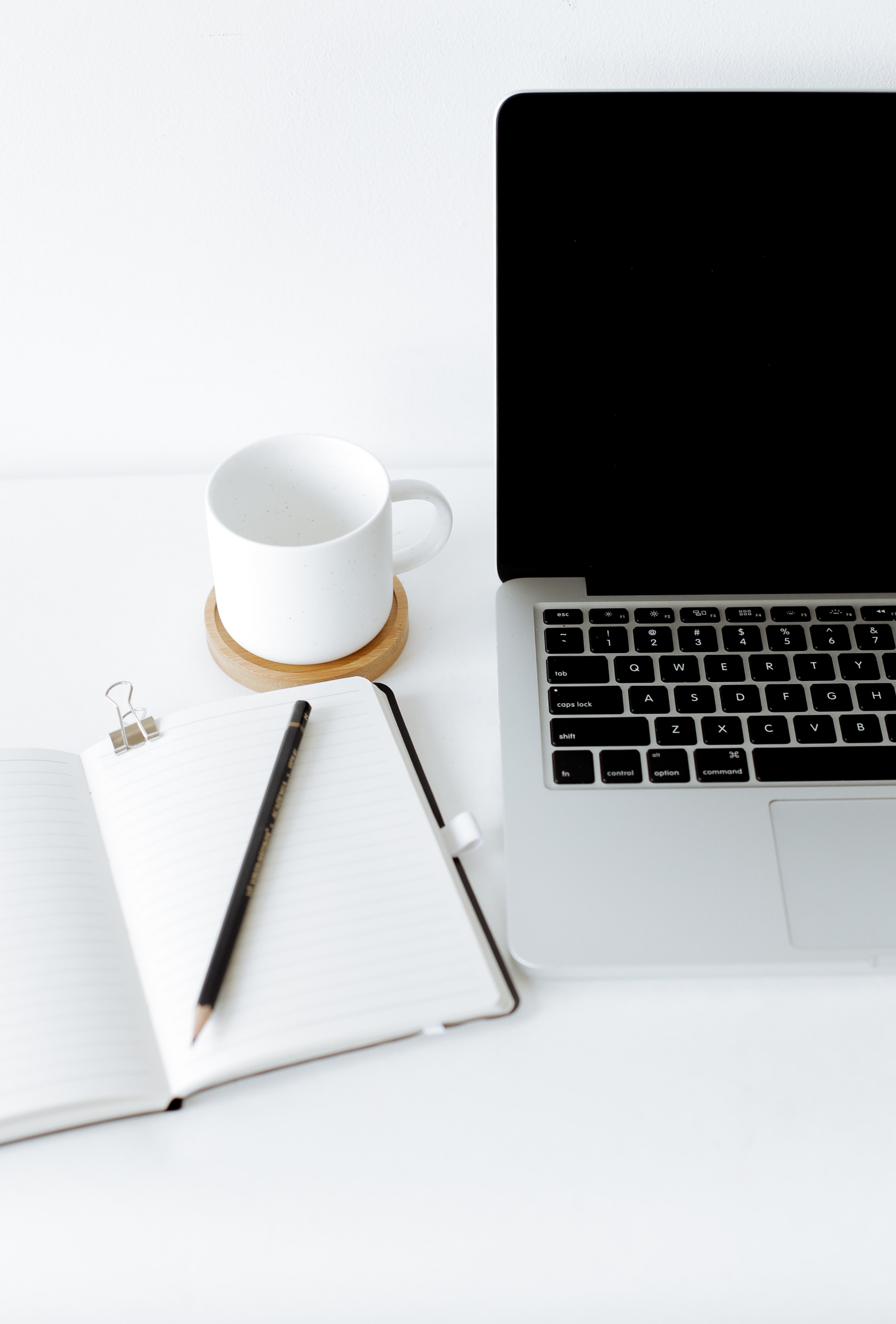